I am a Ph.D. Candidate (2022.08-) in Computing Information & Science here at Rochester Institute of Technology (RIT) supervised by Prof. Weijie Zhao. My research interests span LLMs, AI Privacy & Security, and Scalable & Trustworthy Machine Learning.
Prior to RIT, I worked as a research assistant at the Institute of Computer Vision (ICV), Shenzhen University, China, and was supervised by Prof. Linlin Shen (Honorary Professor at the University of Nottingham, UK). Besides, I used to work as a software engineer (intern) at AI Lab of ByteDance inc. (TikTok’s Parent Company).
I got the bachelor’s degree in Computer Science and Technology in July 2021. I was the leader of a project funded by the National Ministry of Education of China for undergraduates in 2019. During my period of undergraduate, I received an ACM-ICPC Asia Bronze Medal in the ACM-ICPC International Collegiate Programming Contest, and a Silver Medal in the ACM-ICPC Shaanxi Province Contest of China.
My curriculum vitae can be found at here.
Aug. 23, 2024: Invited as a reviewer for ICLR 2025.
Jul. 21, 2024: Invited as a reviewer for ACL ARR 2024.
Jul. 12, 2024: Invited as a reviewer for KDD 2025.
May. 16, 2024: Our paper (Token-wise Influential Training Data Retrieval for Large Language Models) is accepted by ACL 2024! In this paper, we propose RapidIn, a scalable framework for estimating the influence of each training data sample on LLMs.
Apr. 16, 2024: I will be joining Amazon as an Applied Scientist Intern in Boston this summer, focusing on large language models and multimodal systems. 🎉🎉🎉
Feb. 26, 2024: We released an easy-to-run implementation for finetuning large language models (LLMs) such as llama and gemma, supporting full parameter finetuning, LoRA, and QLoRA. Please feel free to star, fork, and make your own contributions. [Github Repo]
Feb. 12, 2024: Invited as a reviewer for KDD 2024.
Oct. 02, 2023: Invited as a reviewer for NeurIPS 2023 GLFrontiers Workshop.
Aug. 05, 2023: Received the KDD’23 Student Travel Award. Thanks to KDD!
May. 16, 2023: Our paper (Machine Unlearning in Gradient Boosting Decision Trees) is accepted by KDD 2023! [Promotion Video, Poster]
Sep. 12, 2022: Our paper (Activation Template Matching Loss for Explainable Face Recognition) is accepted by the 2023 IEEE Conference on Automatic Face and Gesture Recognition (FG 2023)!
Tech Talks
- “Token-wise Influential Training Data Retrieval for Large Language Models” at ACL Virtual Poster Session, Aug. 12, 2024. [Slides, Video]
- “Toward Explainable Large Language Models via Influence Estimation” at Boston, MA, May. 23, 2024. [Poster]
- “Machine Unlearning in Gradient Boosting Decision Trees” at Long Beach, CA, Aug. 9, 2023. [Slides, Video]
- “Activation Template Matching Loss for Explainable Face Recognition” at Rochester Institute of Technology, Nov. 17, 2022. [Slides, Video]
- “Toward Explainable Face Recognition” at Shenzhen University, Oct. 28, 2021. [Slides]
- “Trust in Black-Box Models: Interpretability & Explainability for Deep Learning” at Shenzhen University, Aug. 13, 2021. [Slides]
Selected Publications
- Huawei Lin, Jikai Long, Zhaozhuo Xu, Weijie Zhao, Token-wise Influential Training Data Retrieval for Large Language Models. ACL 2024.
- Huawei Lin, Jun Woo Chung, Yingjie Lao, Weijie Zhao, Machine Unlearning in Gradient Boosting Decision Trees. KDD 2023.
-
Huawei Lin, Haozhe Liu, Qiufu Li, Linlin Shen, Activation Template Matching Loss for Explainable Face Recognition. 2023 IEEE 17th International Conference on Automatic Face and Gesture Recognition (FG), 2023
- Yong Qi, Huawei Lin, Yanping Li, Jiashu Chen, Parameter-free Attention in fMRI Decoding. IEEE Access, 2021.
- Yong Qi, Menzhe Qiu, Huawei Lin, Jiashu Chen, Yanping Li, Huaiguang Lei, Research on Gender-related Fingerprint Features. 2021.
Research Experience
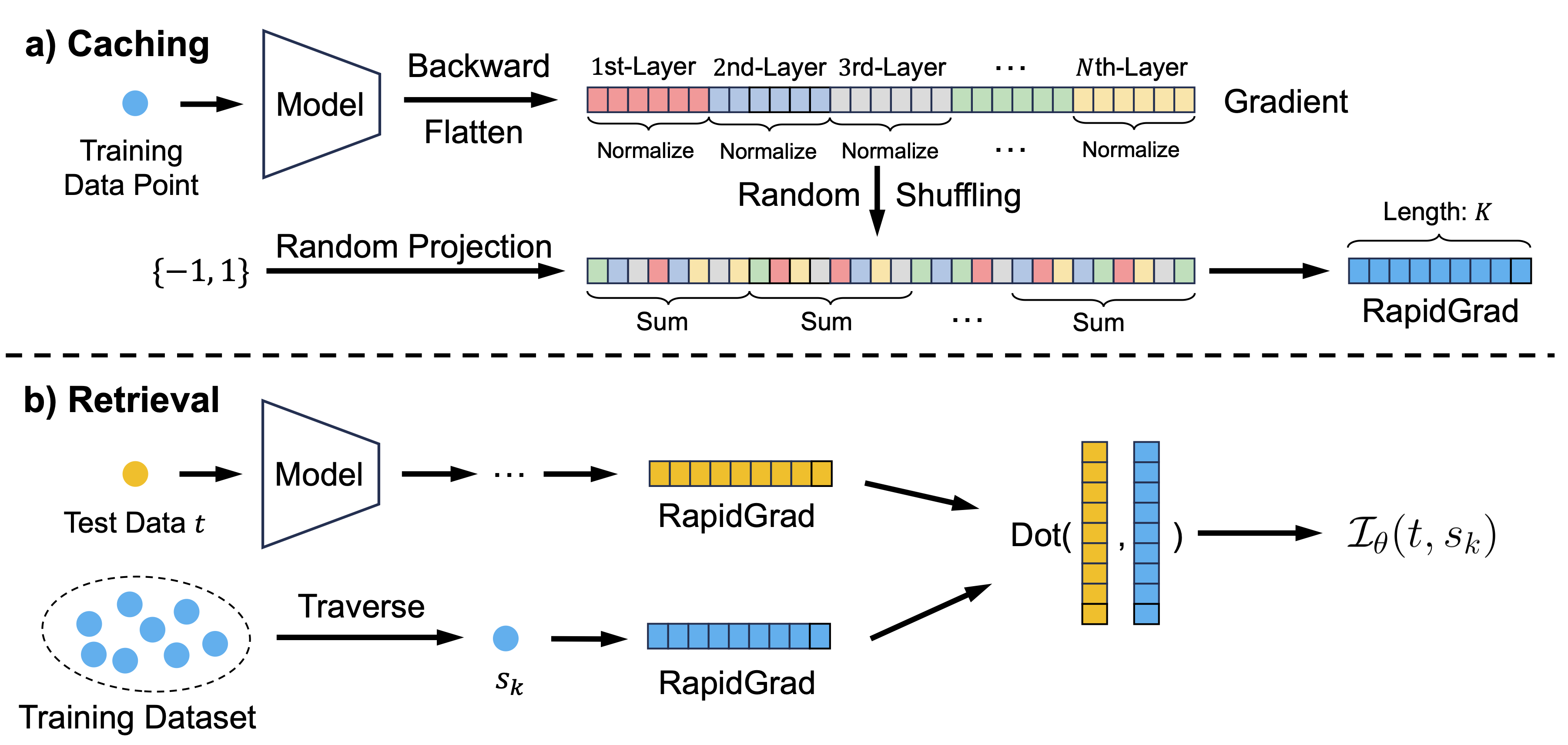
Token-wise Influential Training Data Retrieval for Large Language Models
- Present RapidIn that estimates the influence of each training data for a given LLM generation.
- We apply a collection of techniques to cache the gradients of LLMs by compressing gradient vectors by over 200,000x in the caching stage, and achieve a 6,326x speedup in the retrieval stage, enabling estimating the influence of the entire dataset for any test generation within minutes.
- We utilize multi-GPU parallelization to substantially accelerate the caching and retrieval.
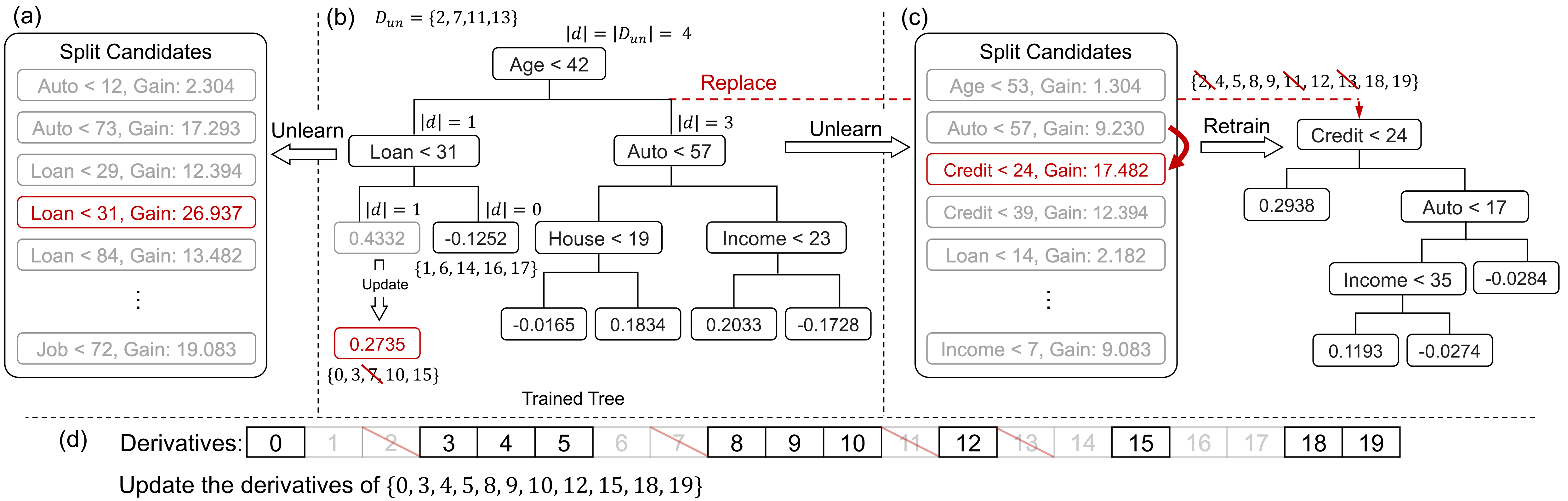
Machine Unlearning in Gradient Boosting Decision Trees (GBDT)
- Propose an unlearning framework that efficiently and effectively unlearns a given collection of data without retraining the model from scratch.
- Introduce a collection of techniques, including random split point selection and random partitioning layers training, to the training process of the original tree models to ensure that the trained model requires few subtree retrainings during the unlearning.
- To the best of our knowledge, this is the first work that considers machine unlearning on GBDT.
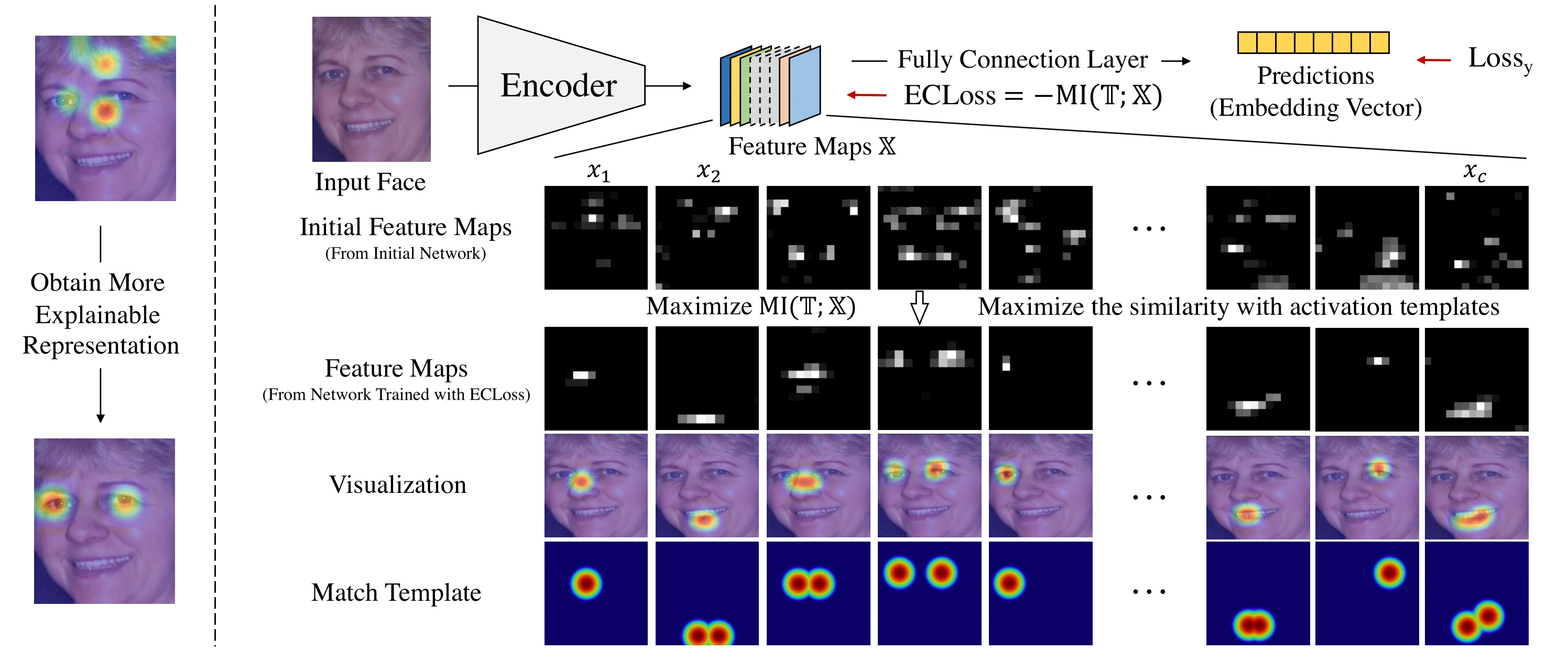
Activation Template Matching Loss for Explainable Face Recognition
- Propose a novel method named Explainable Channel Loss (ECLoss) to construct an explainable face recognition network, which can directly explain that what face recognition networks have learned.
- To the best of our knowledge, this is the first method to construct a feature level explainable face recognition network that does not require any additional dataset or manual annotation.
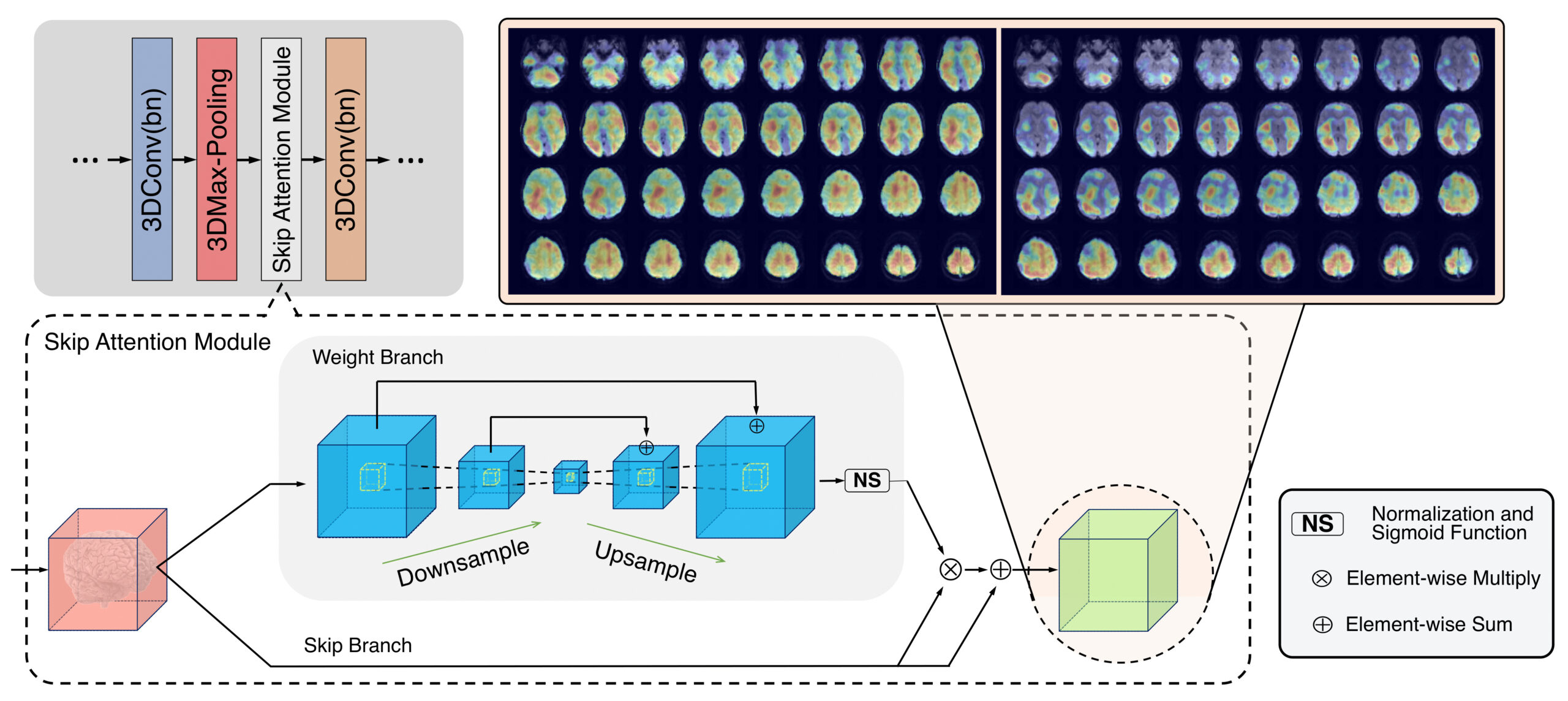
Parameter-free Attention in fMRI Decoding
- Led a team working on a research project that was selected as a National Training Program of Innovation and Entrepreneurship for Undergraduates and funded by the Chinese National Ministry of Education.
- Proposed a parameter-free attention module named Parameter-free Attention Module (SAM) to reduce the average error rate by 1.2%-3.1% while without involving any parameter.
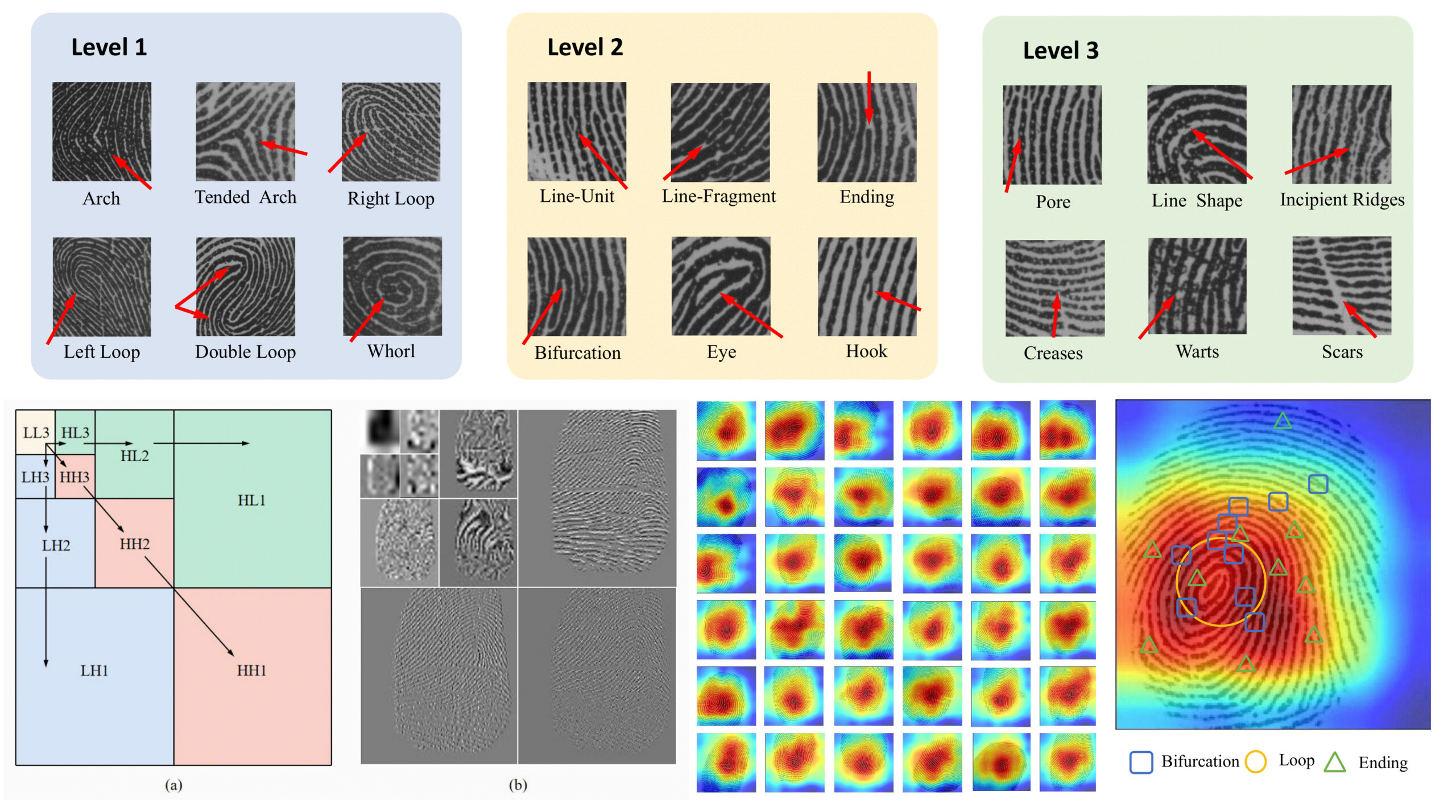
Gender-Related Feature Extraction from Fingerprints
- Designed an architecture called Dense Dilated Convolution ResNet (DDCResNet) to improve the decoding performance of the feature extraction algorithms.
- Achieved an average extraction accuracy of 95%, which significantly exceeds traditional feature extraction methods.
- Improved the interpretability of the algorithms by using Gradient-weighted Class Activation Mapping (Grad-CAM) to visualize the high-score regions of gender information in fingerprint images.
[Paper]
Internship Experience
Amazon, AGI, Boston, MA
May 2024 - Present
Applied Scientist Intern
- Working on Large Language Models and Multimodal LLMs.
Institute of Computer Vision, Shenzhen University, Shenzhen, China
July 2021 - July 2022
Research Assistant | Supervisor: Prof. Linlin Shen
- Responsible for interpretability and explainability on deep learning, especially on Biometrics.
- Propose a novel method named Explainable Channel Loss (ECLoss) to construct an explainable face recognition network, which can directly explain that what face recognition networks have learned.
- To the best of our knowledge, this is the first method to construct a feature level explainable face recognition network that does not require any additional dataset or manual annotation.
AI Lab, ByteDance Inc., Beijing, China
Oct. 2020 - July 2021
Software Engineer
- Responsible for Audio Recognition and Understanding research and development, and technical supports for ByteDance’s applications, including TikTok.
- Proposed Stream Audio Understanding Chain method which enabled real-time audio processing and achieved precise extraction of information from audios, including speakers’ genders, tones, emotions, etc.
- Designed a pipeline processing flow that significantly increased the throughput of CPUs and reduced processing time by 65%, by optimizing the usage of CPUs/GPUs using multiprocessing and multithreading.
Fellowships & Awards
Fellowships
- KDD23, Student Travel Awards Aug. 2023
- Zhou Lian Academic Scholarship (Only 1 of ~20,000 Students) Oct. 2020
- Academic Innovation and Technology Scholarship (Only 10 of ~20,000 Students) May 2020
- IAPR/IEEE Winter School on Biometrics 2020, Student Travel Grants Jan. 2020
- Academic Innovation and Technology Scholarship (Only 10 of ~20,000 Students) May 2019
Awards
- ACM-ICPC National Programming Contest (Shaanxi), Bronze Medal June 2021
- ACM-ICPC Programming Contest (Shaanxi Province), Silver Medal Sept. 2020
- ACM-ICPC National Programming Contest (Shaanxi), Bronze Medal June 2020
- ACM-ICPC National Programming Contest (Yinchuan), Bronze Medal May 2019
- ACM-ICPC Asia Regional Contest, Bronze Medal Nov. 2018
- ACM-ICPC Chinese Collegiate Programming Contest, Bronze Medal Jan. 2018
Professional Services
Reviewer
- ICLR 2025
- ACL ARR 2024
- KDD: 2024, 2025
- NeurIPS 2023 GLFrontiers Workshop
Open Source Project Contribution
We have a team dedicated to building open-source AI systems about Artificial General Intelligence (AGI), Generative AI (GAI) and Large Language Models (LLMs). [Website, GitHub]
TECHNICAL SKILLS
- Programming Languages: C/C++, Python, Go, Java, Shell, HTML
- Deep Learning Tools: PyTorch, CUDA, Keras, TensorFlow
- Deep Learning Packages: Transformers, DeepSpeed, FSDP, PEFT, OpenAI
- Others: Slurm, MATLAB, Docker, Hadoop, Kubernetes, Kafka